Last month Dilfuza successfully defend her PhD dissertation from the questions of her
two examiners: Ana Rita Brochado and Dan Depledge.
Congratulations to her for pulling this off and be the first PhD student to graduate from our lab!

We are very happy to report that the first PhD thesis from the lab has been submitted this month!
With just one day to spare before the deadline (as it should be đ
), Dilfuza has submitted her
thesis to the ZIB office. Now we wait for the public defense in June.
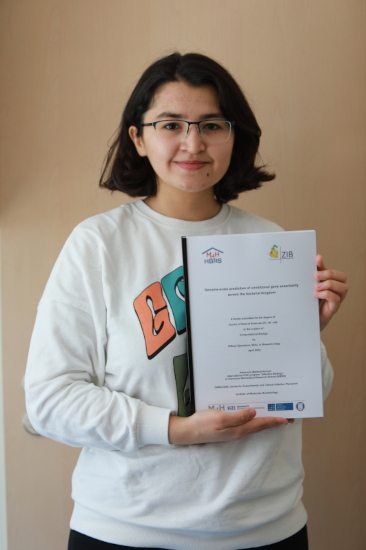
In other news, last month Adam has officially left the lab to take an exciting new job as a postdoc
in the lab of Craig MacLean at the University of Oxford. Luckily Adam made a big push
before leaving and finished some large scale experiments thanks to his usual stamina, which will be
missed!
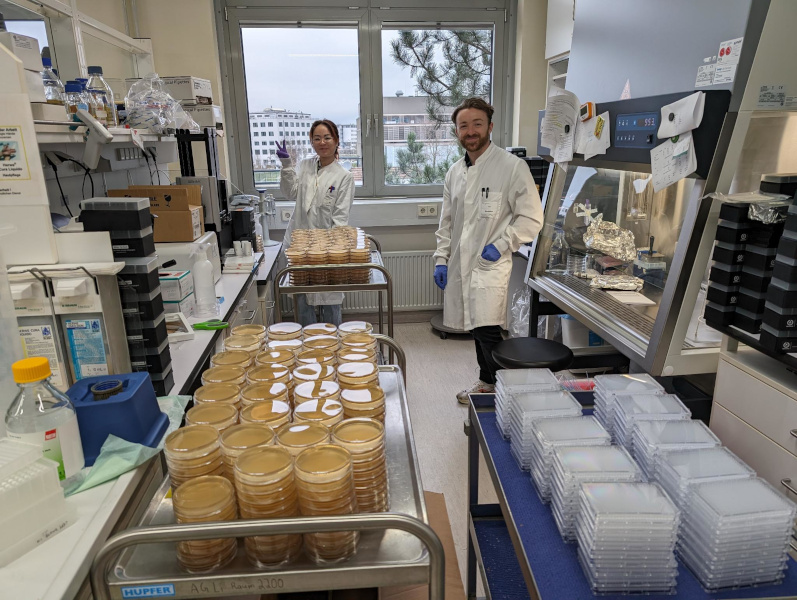
Congratulations to Dilfuza and Adam for these exciting news!
As anticipated in the previous post,
we intended to run the Hannover half marathon, and indeed we did!
We all managed to get to the finish line, with a spatial mention to Hannes, who completed
the race in 01:59:52, with just 8 seconds to spare for the 2-hour psychological
barrier!
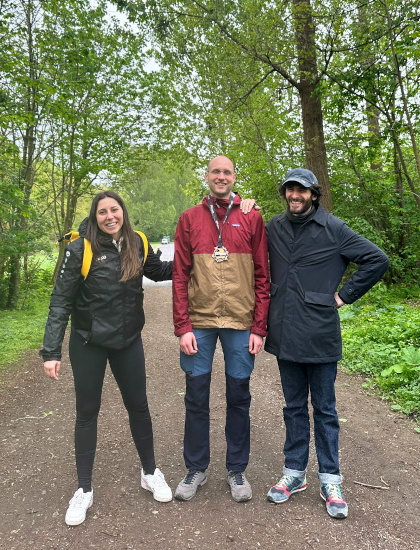
We didnât quite manage to get a photo on the day of the race, but we are happy to report
that we collected 366 Euros through our DKFZ donation campaign. Thanks to all who donated!
Our lab (Hannes, Judit and Marco) is running the Hannover half marathon on the 14th of April,
and we have decided that in order to boost our determination we needed support from colleagues, friends, and family.
And what better way to do it but to collect donations for a worthy cause? As researchers ourselves,
we have decided to support cancer research through the DKFZ (the German Cancer Research Center).
Cancer is the second cause of death in Germany, with an estimate ~270â000 deaths in 2019. Improved diagnostics and
therapeutics have reduced the death rates from cancer since the 90s, which clearly proves how a donation to cancer research has
the potential to affect the lives of many patients. So please help us get through the last two weeks of training and
through race day by supporting Cancer research! Follow the link below and scroll to the bottom of the page to find
the donation button. Thanks!
The Microbial Pangenomes Lab is running a Half Marathon for Cancer Research - DKFZ
P.s. if you are having trouble getting through the payment system do get in touch with Marco, and he can make
the donation on your behalf and collect the money afterwards through a bank transfer.
Just a couple of days ago we have published a research article describing
panfeed, a software tool
to aid bacterial GWAS studies. This effort was
led by Hannes, with the help of Dilfuza
during peer review.
As everyone in the field of genomics has heard ad nauseam, we now have an abundance of
genome sequences available; when that is combined with phenotypic measurements the obvious
question is then âwhich gene is responsible for this phenotype?â. Statistical genetics (i.e.
genome-wide association studies, GWAS) would be one way to answer that question, or rather
the more correct one âwhich genetic variant is associated, and hopefully causal, for the
variation in phenotype, across this collection of genomes?â.
When asking this question in the context of bacterial genomes, the term âgenetic variantâ
can take a number of meanings, going from âclassicalâ short variants such
as SNPs/InDels, to entire operons being transferred horizontally and even genes changing
their arrangement. One clever way to
encode all these variant types is to use a k-mer, which is a DNA âwordâ of length k
(typically 31).
While this solution allows one to collapse different genetic variant types into a unified
data structure, it generates two problems: one of ambiguity and one of interpretability.
These are the problems that we aim to ameliorate (hopefully solve for most people!) with panfeed.
Letâs go over both problems then.
Greatly simplyfing, one way to generate k-mers from a set of genomes is to take the input genomes
and put them into a blender that chops them into k-mers. The output can be then seen as a big haystack
from which we have to find the proverbial needle (the causal variants). The problem is that some genes
(which in bacterial genomes are pretty much the main unit of molecular function) will share some k-mers,
which will then implicate unrelated genes with the focal phenotype (as well as subtly modifying the presence/absence patterns
of those shared k-mers, affecting the association results themselves). Thatâs the ambiguity problem that needs to be solved;
using data from 21 bacterial speciesâ genomes we indeed show how on average 3% of k-mers are duplicated across genes
and how 13% of genes have at least one k-mer shared with at least another gene.
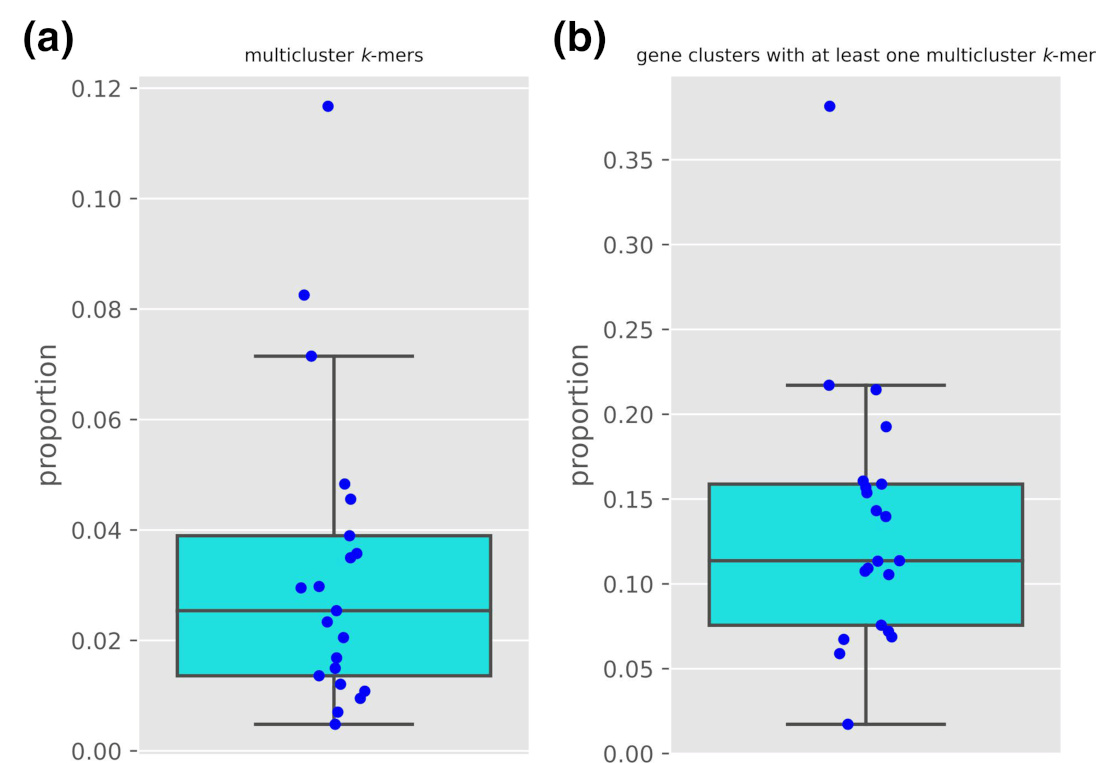
The problem of interpretability also stems from all genetic variants being in a big haystack: once you have found your needle,
how do you figure out 1) which gene it might belong to, and 2) which variant type it encodes?
You can surely map the k-mers back to the input genomes, but you would lack the local context, meaning which other
genetic variants are present in that genomic location across all the genomes in the sample.
We address both problems by leveraging the assumption that genes are the unit of function in bacterial genomes and what
most people will use to interpret results. So basically instead of having a single haystack, we generate many (tens of thousands in fact!)
smaller ones and search for a needle in each of them. To be more specific, we use the gene cluster definitions given by tools
such as panaroo and ggCaller
and extract k-mers and their presence/absence patterns from each gene cluster separately.
This means that a k-mer that is duplicated across gene clusters is allowed to have a different presence/absence pattern for each gene,
thus reducing false gene leads.
Interpretation is improved by linking k-mers and their source gene directly, as well as showing the local context of the associated variants,
which can optionally include promoter regions, as shown below.
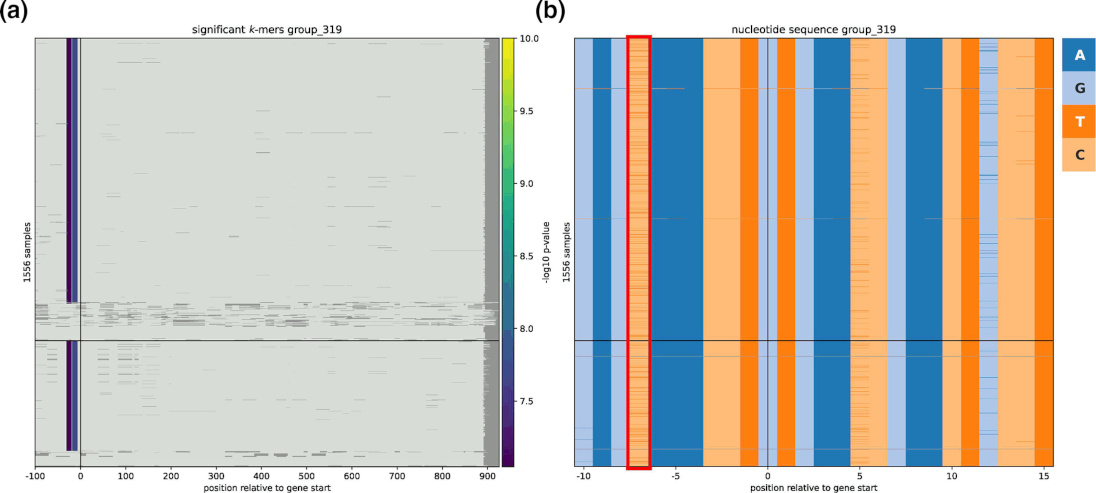
panfeed is available as a conda package in bioconda (mamba create -c bioconda -n panfeed panfeed
),
as a pypi package (python3 -m pip install panfeed
),
and its source code and issue tracker can be accessed in our labâs github. Happy needle search!